Here's How The Channel Can Follow The Data To Bigger Revenue
Submitted by Jason Huling on
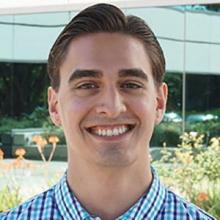
The Power Of Prescriptive Analytics
One of the most interesting forms of analysis that has been gaining momentum recently is called prescriptive analytics. In the scenario of a sales campaign in which you’re trying to increase service revenue, a simplified example of how you would run this analysis would be to build four service contract quotes and apply a different discount to each, while monitoring the effect of the discount on the end user’s probability to buy. If we have a $100 quote and start out with no discount, we observe that there is a 30 percent probability that the user will purchase the quote. Next, we offer a $10 discount and the probability doubles to 60 percent. We then look at a $20 discount, which results in an 80 percent probability to purchase. Finally, we analyze a $30 discount, which only sees a 5 point increase to an 85 percent chance to buy.
Every reseller will view these results differently depending on their goals and priorities, with some putting more emphasis on the highest probability to buy, while others will focus on the dollar value of each quote. While this is a very small example of the general concept, the beauty of machine learning is the ability to take into account a nearly limitless number of scenarios. Instead of just four service quotes with different discounts, it could be hundreds to thousands of possible quotes for a single end client. Each quote in your analysis would have a different combination of attributes, such as applied promotions; various service levels; hardware refresh suggestions; and even suggested involvement by a sales rep. These combinations are then ranked, based on key values for the campaign, to determine which quote to ultimately create and deliver. And these key values can include items such as the client’s probability to purchase, and the revenue that can be realized if the quote is purchased, keeping in mind that these keys and weights can differ from campaign to campaign.
Get Started
As you can see, this iterative and scenario-driven approach can be very powerful for delivering the best service quotes and offers to your customers. It’s important to remember, however, that using data science in sales is only possible when you have the necessary data available, and the tools and resources needed to analyze it. And like everything else in business, without reporting you can’t effectively track results and key performance indicators – not to mention plan and strategize to improve future outcomes in service sales. It’s always critical to know what’s working, what isn’t and how you can make best use of your BI and your resources.
Whether you outsource to get where you need to be with data science or rely upon the support and guidance of the distributors and manufacturers that you’ve partnered with, the important thing is to get started. Investing in data science as part of your service revenue practice will give you new-found precision in reaching out to your customers with timely service offers, and it has been proven to lead to substantial revenue gains as well. In the end, it will also help you keep a better pulse on customers by allowing you to gain valuable insight into their interests and preferences, both now and into the future.